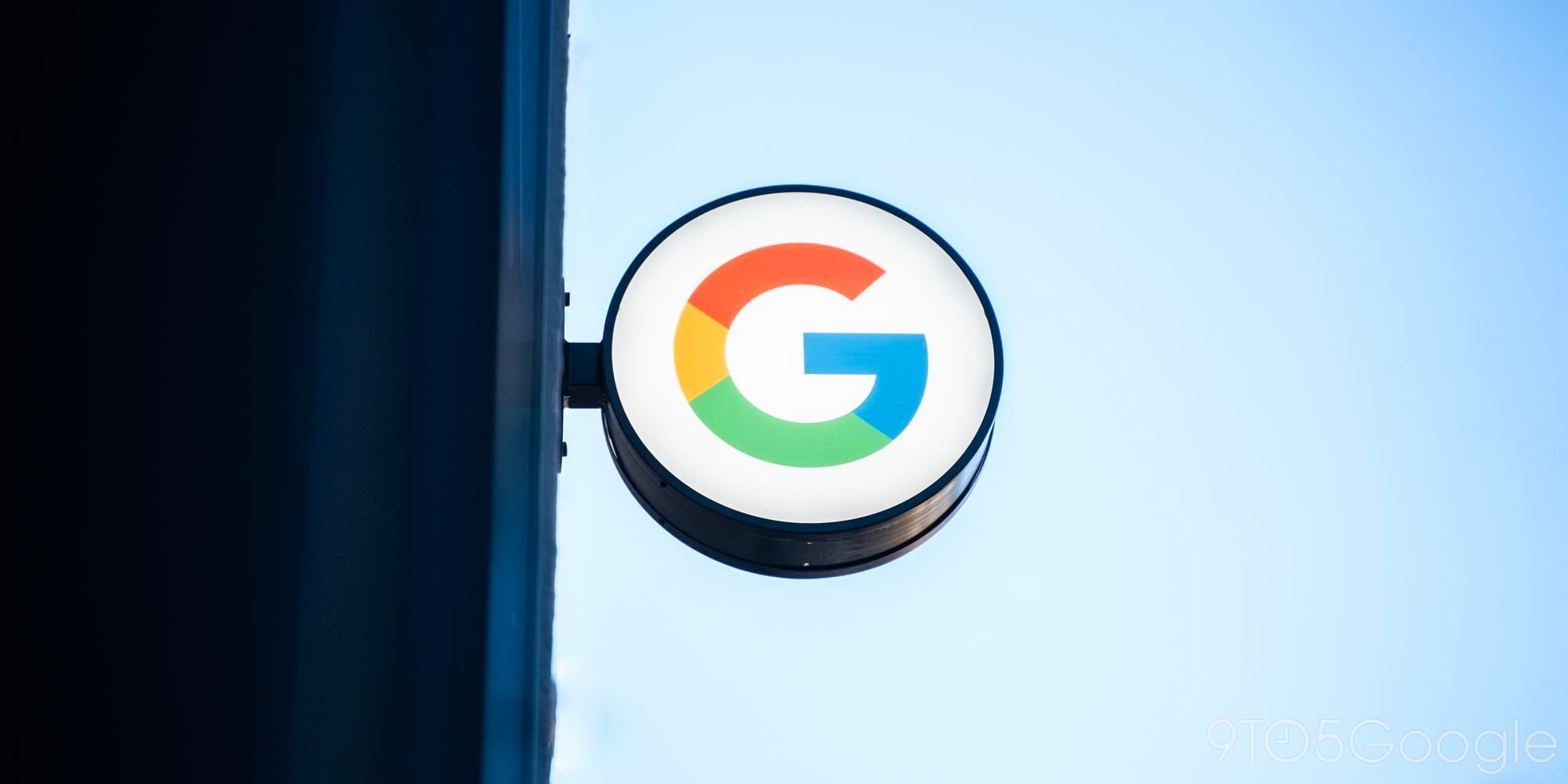
Machine learning is increasingly being leveraged in a variety of consumer products and other research. The latest use sees Google apply AI to planet hunting and in the process discovering two previously overlooked exoplanets.
Since its launch in 2009, NASA’s Kepler Space Telescope has been tasked with scanning the night sky for exoplanets. Over a four-year period, the spacecraft has observed 200,000 stars and captured an image every 30 mins, resulting in 14 billion data points.
In turn, that results in 2 quadrillion possible planetary orbits. Conventionally, astrophysicists use automated software and even manual review to discover new exoplanets that orbit a star outside of our own solar system.
As part of a 20 percent project, one Google AI researcher decided to use machine learning “to make this process faster and more effective.”
Using a dataset of more than 15,000 labeled Kepler signals, we created a TensorFlow model to distinguish planets from non-planets. To do this, it had to recognize patterns caused by actual planets, versus patterns caused by other objects like starspots and binary stars.
Testing this model on new data, the system correctly identified which signals were planets and which were not with a 96 percent accuracy rate. Following this training, Google — along with an astrophysicist from UT Austin — used the system to hunt new planets.
With 200,000 stars in Kepler’s data, the team had the model look at 670 stars that had two or more previously discovered exoplanets. In the process, they discovered two new planets: Kepler 80g and Kepler 90i.
Significantly, Kepler 90i is the eighth planet discovered orbiting the Kepler 90 star, making it the first known 8-planet system outside of our own.
The duo plan to apply the model to the rest of Kepler’s data.
FTC: We use income earning auto affiliate links. More.
Comments