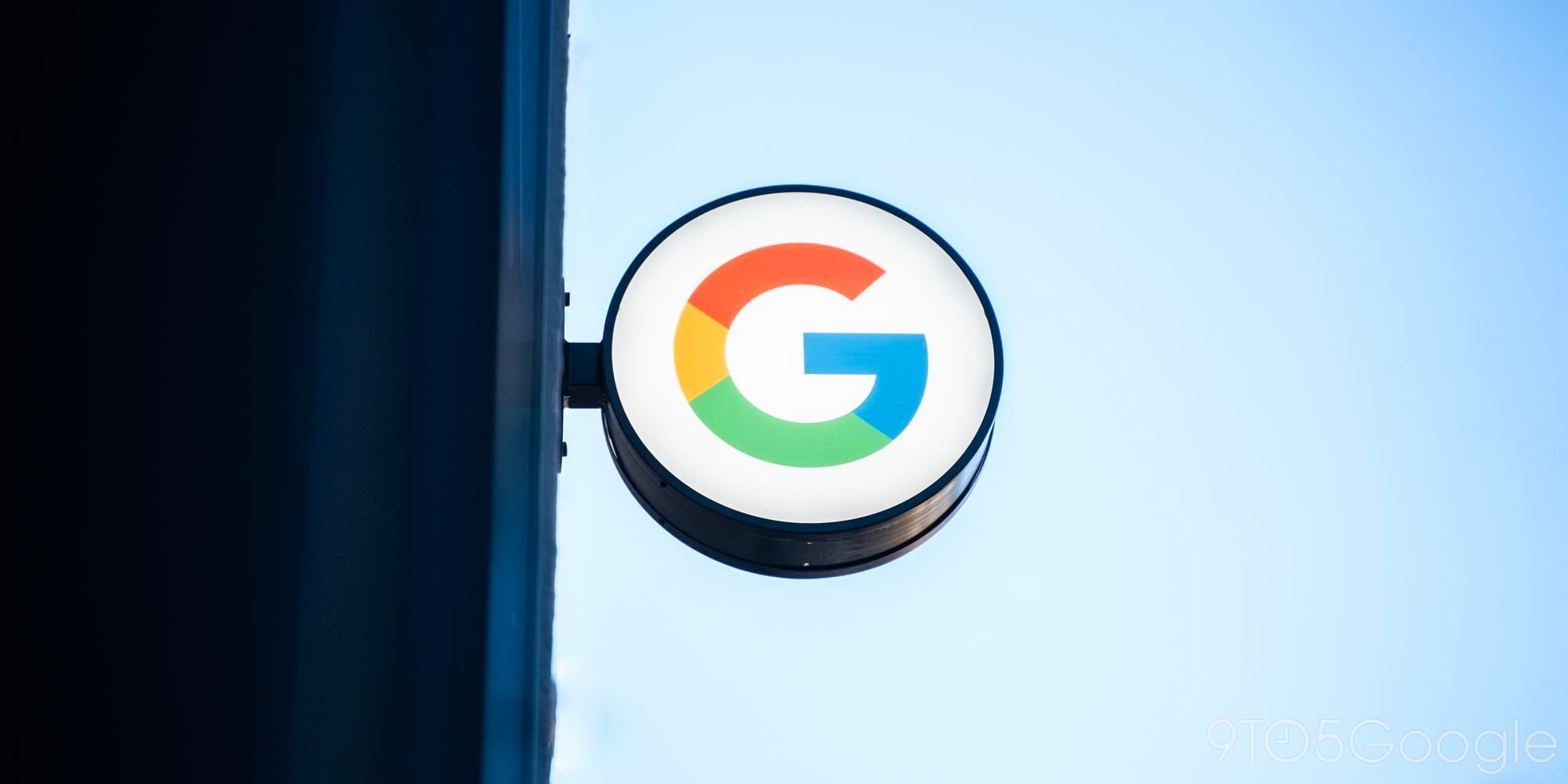
One of the first applications of DeepMind at Google was to reduce and now control power usage at its data centers. Google is now leveraging machine learning to make more efficient wind farms by predicting wind power output 36 hours in advance.
The two Alphabet divisions worked together to train a neural network on weather forecasts and historical turbine data. A DeepMind system was then able to “predict wind power output 36 hours ahead of actual generation.”
By reducing the variability of wind power, the renewable energy source becomes “sufficiently more predictable and valuable,” giving wind farm operators more data-driven assessments of how to meet upcoming electricity demand.
Based on these predictions, our model recommends how to make optimal hourly delivery commitments to the power grid a full day in advance. This is important, because energy sources that can be scheduled (i.e. can deliver a set amount of electricity at a set time) are often more valuable to the grid.
The algorithm is still being refined, but Google notes how machine learning — compared to no time-based commitments to the grid — has “boosted the value of our wind energy by roughly 20 percent.” The company is applying this optimization to its wind farms in the central United States that generate 700 megawatts of wind power.
In practice, Google wants to make wind power a better and more valuable source of renewable energy by integrating machine learning. Better ML-backed predictions about wind power production and electricity supply/demand help create operational cost savings. Elsewhere in Alphabet, Makani is working with Shell to deploy wind kites offshore that are light and more versatile than wind turbines.
FTC: We use income earning auto affiliate links. More.
Comments